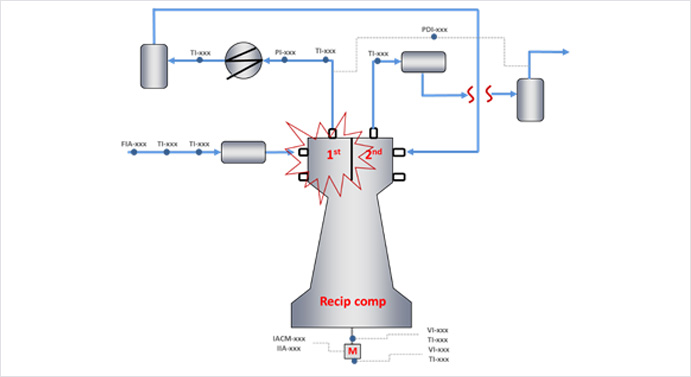
Case Study
Anomaly Detection for Valve Plate in Vertical Reciprocating Compressor Damage Using Machine Learning Model
CLIENT :
N/A
SERVICE :
Reliability Enhancement
The Situation
Our client experienced low reliability of the multi-stage vertical reciprocating compressor due to unforeseen suction valve plate damage, an internal part used to control the direction of compressed gas flow. Although online vibration analysis and automatic fault notification were in place, it can detect only severe damages, which can lead to both production interruption and high maintenance cost.
By looking at historical data from the machine’s process and parameters, a pattern emerges that shows gradual changes in the machine’s operation. The pattern becomes more noticeable as the vibration becomes increasingly severe. Unfortunately, continuous monitoring of all parameters related to this problem by human is impractical and a more advanced approach is required.
Our Approach
A predictive analytic model using Machine Learning (ML) was introduced to effectively solve this problem. A pattern recognition model was applied to memorize all the patterns from various parameters such as flow, temperature, pressure, and vibration during the normal health condition. Therefore the model can identify problems immediately in the case of an emergent of a different pattern.
In the case of valve plate damages, domain knowledge is essential for parameter selections and deviation patterns, or fault diagnostic, which are essential in the early stages of damage. The model developed by our data scientist was based on this domain knowledge so that it can deliver;
- Early anomaly detection, which is about 30 days before high vibration was introduced
- Suggested solution, also called prescription
Apart from the the valve plate issue, the combination between the domain knowledge and data science approaches can be applied for the other possible failures so that asset reliability can be guaranteed.
Value Delivered
- Guarantees zero unplanned breakdown.
- Minimizes loss during maintenance period.
- Utilizes existing sensor data which is an essential approach for predictive maintenance capability enhancement.
- Can be applied to other assets.
Reference :
- J. W. Hines, J. Garvey, D. R. Garvey, and R. Seibert, "Technical Review of On-Line Monitoring Techniques for Performance Assessment Volume III: Limiting Case Studies," The Office of Nuclear Regulatory Research, 2008.
- K. C. Gross and W. Lu, "Early detection of signal and process anomalies in enterprise computing systems," in the 2002 IEEE international conference on machine learing and applications (ICMLA), Las Vegas, 2002.
- P. Baraldi, R. Canesi, E. Zio, R. Seraoui, and R. Chevalier, "Signal grouping for condition monitoring of nuclear power plant components," in Advances in Safety, Reliability and Risk Management: ESREL 2011, 2011, pp. 1-13.